What will the future look like of medicine with artificial intelligence?
by admin
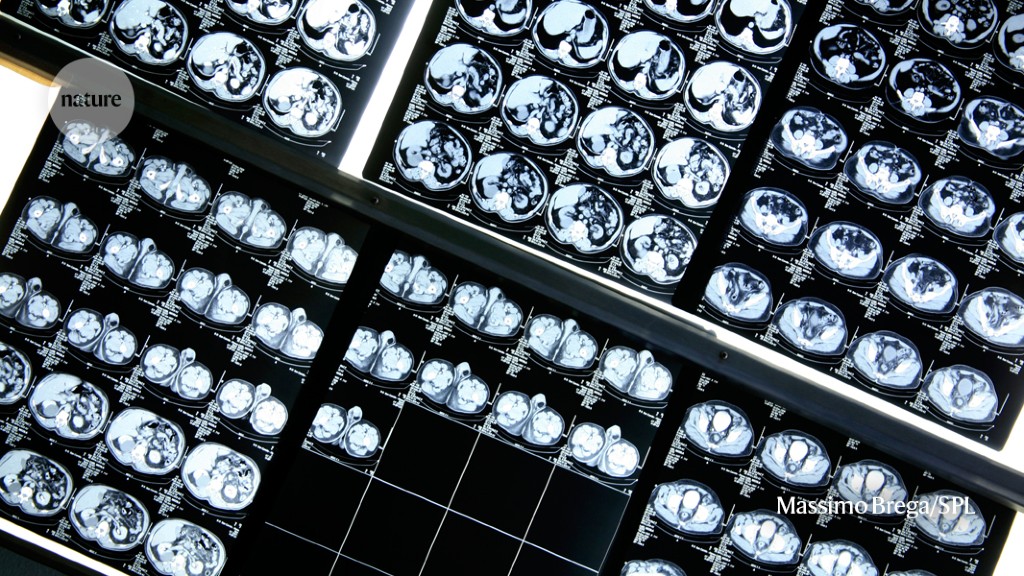
When Artificial Intelligence Meets Physicians: An Empirical Case Study of a Patient Whose Breathing Is Alive and Well
It is possible to use artificial intelligence tools to provide support for practitioners, such as going through scans quickly and spotting issues that a doctor might want to look at immediately. Sometimes the tools work well. Someone was flagged for a chest ct scans by analyst when they were having difficulty breathing. The middle of an overnight shift was 3 a.m. He agreed to the assessment that the Scan showed a pulmonary embolism that required immediate treatment. Had it not been flagged, the scan might not have been evaluated until later that day.
But if the AI makes a mistake, it can have the opposite effect. Perchik says he recently spotted a case of pulmonary embolism that the AI had failed to flag. He decided to take extra review steps, which confirmed his assessment but slowed down his work. If I had just decided to get on with it, that could have gone undetected.
If you scale that to a health system, you can see the choices you have to make when it comes to devices and how to integrate them. It can quickly become an IT soup.
The foundation of medical artificial intelligence: a case study in eye disease detection from retinal photos and scans by Pearse Keane
It is better known in the field that you need to do an external validation. But, she adds, “there’s only a handful of institutions in the world that are very aware of this”. Without testing the performance of the model, particularly in the setting in which it will be used, it is not possible to know whether these tools are actually helping.
Aiming to address some of the limitations of medicine’s artificial intelligence tools, researchers are exploring broader capabilities of medical artificial intelligence. They’ve been inspired by revolutionary large language models such as the ones that underlie ChatGPT.
Some scientists call these examples of a foundation model. The term, coined in 2021 by scientists at Stanford University, describes models trained on broad data sets — which can include images, text and other data — using a method called self-supervised learning. Base models are pre trained models that can be used to perform different tasks.
Foundation models have no requirement for the annotation of large numbers of images. For ChatGPT, for example, vast collections of text were used to train a language model that learns by predicting the next word in a sentence. Similarly, a medical foundation model developed by Pearse Keane, an ophthalmologist at Moorfields Eye Hospital in London, and his colleagues used 1.6 million retinal photos and scans to learn how to predict what missing portions of the images should look like4 (see ‘Eye diagnostics’). The researchers introduced a few hundred labelled images to the model that were used to learn about certain sight-related conditions. The system was better than previous models at detecting these ocular diseases, and at predicting systemic diseases that can be detected through tiny changes in the blood vessels of the eye, such as heart disease and Parkinson’s. The model has not been tested in a clinical setting.
Big tech companies have invested in medical-imaging foundation models that use multiple image types and incorporate electronic health records.
Scientists are optimistic that the models might be able to identify patterns that humans can’t. Keane mentions a 2018 study by Google researchers that described AI models capable of identifying a person’s characteristics — such as age and gender — from retinal images8. That is something that even experienced ophthalmologists can’t do, Keane says. There is a real hope that there is a lot of scientific information in these high-dimensional images.
A study published in the Journal of Medical Internet Research in August tested out the diagnostic skills of the popular ChatGPT program. When making diagnoses, the artificial intelligence program was 77% accurate according to the researchers. With more limited information based on patients’ initial interactions with doctors, though, ChatGPT’s diagnoses were just 60% accurate.
The transplant specialist at Massachusetts General Hospital hopes that the invention of artificial intelligence will allow him to spend more time with patients. He says that if you would allow him to go and talk to that person about their diagnosis, he could spend less time searching for things. “It restores that patient-doctor relationship.”
“AI won’t replace doctors, but doctors who use AI will replace doctors who do not,” Succi says. “It’s the equivalent to writing an article on a typewriter or writing it on a computer. It is the level of leap.
“It needs improvement,” says Dr. Marc Succi of Mass General Brigham, who was one of the paper’s authors. It’s necessary to improve certain parts of the clinical visit before it’s ready for prime time.
“It’s a time-consuming and very haphazard process,” says Dr. June-Ho Kim, who directs a program on primary care innovation at Ariadne Labs, which is a partnership of Brigham and Women’s Hospital and the Harvard T.H. Chan School of Public Health. Natural language summaries of it being incredibly useful, that’s because a large language model is able to digest it and produce them.
He once saw it cite a journal article in his area of expertise that he wasn’t familiar with. “And I then looked to see if I could find the study in that journal. It did not exist, says Bonis. My next query was to the model, “Did you make this up?” It said yes.”
The AI Helping Doctors Make Better Diagnoses UpToDate: “It’s a Google Drive,” says Dr. Peter Bonis
At this point, Wolters Kluwer Health is just sharing the AI-enhanced program in a beta form for testing. Bonis says the company needs to make sure it’s entirely reliable before it can be released.
“If you have a question, it can keep the context of that question,” says Dr. Peter Bonis. “And saying, ‘Oh, I meant this,’ or ‘What about that?’ And it knows what you’re talking about and can guide you through, in much the same way that you might ask a master clinician to do that.”
“And I get things like dengue virus, jellyfish stings, murine typhus, etc.,” he says, scrolling down a long list of responses on his screen. “I wish the list could have been more specific, I think Genai gives you the opportunity to really refine that”, says Mansour.
“Here’s an example,” Mansour says, turning to his computer. If I meet a patient who is from Hawaii. The hypothetical patient’s symptoms make Mansour worry about an infection that the patient acquired back home, so he types “Hawaii” and “infection” into UpToDate.
Basically, it’s a search of a huge database of articles written by experts in the field, who are all pulling from the latest research.
Source: AI could help doctors make better diagnoses
UpToDate: Up to Date for Fungal Infections in Transplant Patients Using Mansour’s Companion Program UpTodate
When a patient comes in with a mysterious infection, Mansour turns to a computer program called UpToDate. There are more than 2 million users at 44,000 health care organizations in over 190 countries.
Mansour specializes in invasive fungal infections in transplant patients. “Got a nice picture of the mushrooms in my office,” Mansour says with a laugh. “Mold and yeast infections can be very devastating, and I like to help patients through that.”
An ophthalmologist at Moorfields Eye Hospital in London has developed an artificial intelligence (AI) model that predicts what missing portions of retinal photos and scans should look like. Pearse Keane and his colleagues used 1.6 million retinal photos and scans to learn how to predict what missing portions of the images should look like. The model is capable of identifying a person’s characteristics.
Recent Posts
- If you test these techniques outside of the lab, you can see that chemicals can be destroyed with clever chemistry
- A human atlas has a continuous tissue axis
- Researchers receive an updated look at the Human Cell Atlas, and it is remarkable
- There is a lot of off-brand oral ozompic for sale online
- Robert F. Kennedy Jr. has been nominated by Donald Trump toOversee US Public Health