The recovery of freshwater fauna in Europe has stopped
by admin
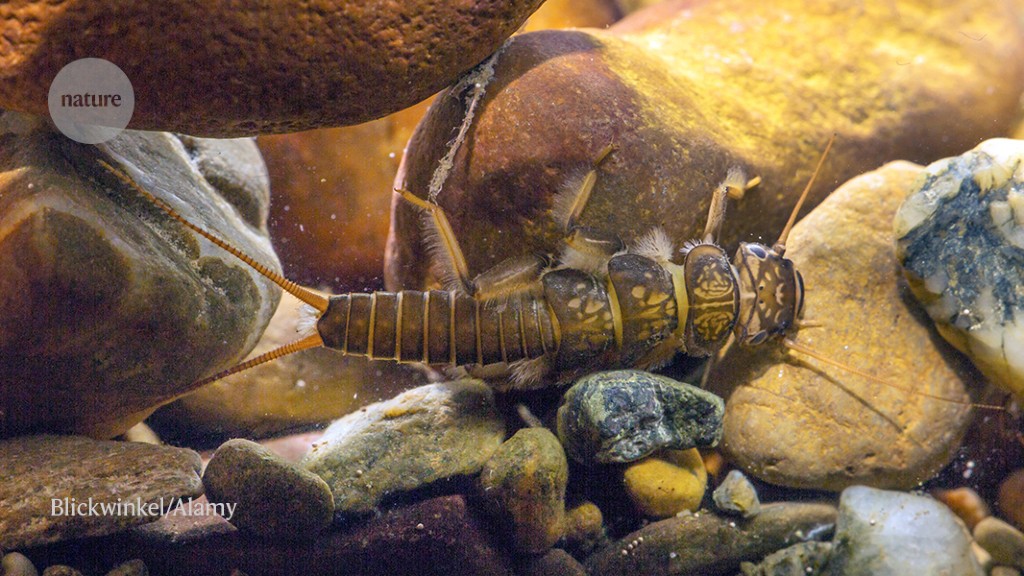
The effects of climate on biodiversity in the bed of the river using a large mixed-effect Bayesian analysis and a synthesis approach
The level of biodiversity is disproportionately high in rivers and other freshwater environments that cover less than 1% of Earth’s surface. There is evidence that they are among the world’s most threatened ecosystems, with some of the largest reported declines in biodiversity2, linked to a combination of factors, such as intense exploitation by humans, pollution and the changing climate. However, a few studies have found evidence of increasing invertebrate biodiversity3. Fortunately, the bed of the river can often be used as a sensitive indicator of river quality, thanks to the native insects that live there. The result is a mixed picture of how river quality has changed over the course of the last three decades, but it’s not entirely clear. Many of the rivers are in a poor condition, and the recovery from historical impairment appears to have run out of steam.
In Bayesian models, we mostly used default brms settings, including four chains, which were run for 5,000 iterations (50% burn-in). We used default priors except for trend estimates, for which we selected a narrower prior to diminish the influence of biologically unrealistic trend estimates. We used normally distributed priors with a mean of zero and an s.d. of 10 or 3 for our site-level trends. We compared our meta-analysis model of trends with and without including the uncertainty of site-level trend estimates. The models used were unweighted for non-native abundance and EPT taxon richness. Functional turnover was fitted using beta models as values were bound between 0 and 1. The percentage change per year was calculated by back-transforming model estimates. Back-transformation calculations varied according to the originally modelled transformations of response variables (see the ‘equationsToPercChangePerYr.xlsx’ file in the ‘plots/Fig2_DensityPlots’ folder at https://github.com/Ewelti/EuroAquaticMacroInverts). We further tested a one-stage synthesis approach in which mean trends were estimated in one large mixed-effect model of the observed data, including random intercepts and slopes. The models produced the same trend results, as seen in figure 16 in the Online Figures.docx file.
The responses of biodiversity metrics to climate were assessed, along with the land cover and dam impact score. We did not include upstream land-use trends as most sites exhibited low variation: cropland cover changed by a mean of −0.002% per year ± 0.11 s.e.m., with no change detected at 634 sites; urban cover changed by 2.48% per year ± 0.14 s.e.m., but with no change detected at 803 sites. To examine relationships between environmental drivers and biodiversity trends, we modelled trend estimates using an LMM, incorporating trend errors as for the calculation of the overall trend, including all predictor variables as fixed effects, and study identity and country as random effects.
A linear mixed-effects model for the estimation of moving window trend estimates. I. Site-level data and mean trends with normal error assumptions
The form of the model was: brm(MovingWindowTrend|se(sd_trend_estimate) ~ year, data = moving_window_trends, iter = 5000, inits = 0, chains = 4, prior = c(set_prior(“normal(0,3)”, class = “Intercept”)), control = list(adapt_delta = 0.90, max_treedepth = 12)).
We used brms80 to model how the year might affect moving-window trend estimates.
For each metric, we took the proportion of the distribution of the mean trend estimate above or below zero to calculate the probability of a rising or falling mean trend.
We ran linear mixed-effects models (LMM) in the brms package to synthesize site-level data and estimate overall mean trends. The LMM included site-level trend estimates as the response, and an overall intercept and two random effects (country and study identity) as predictors. These random effects accounted for data heterogeneity due to unequal numbers of sites among studies and countries. We assumed normal errors since site-level trends were normally distributed. The uncertainty of the estimates of the mean trend was represented by brms 80, used to estimate the site level trends.
Normally distributed errors were assumed to have been checked visually. Taxonomic evenness, total abundance and subset abundance were log10-transformed to meet the normality assumption.
Source: The recovery of European freshwater biodiversity has come to a halt
Land cover and subcatchment proportions for 1992-2019, data analysis and dam impacts prediction from the TerraClimate dataset 79 for 1967-2020
Year in the temporal autocorrelation term was included as a count in the first year of sampling to improve model convergence. The models accounted for any residual temporal autocorrelation with an ar(1) term, as well as the day of year when the variation in sampling dates was greater than 30 days.
We calculated the proportion of land cover categories in each subcatchment using the ESA CCI Land Cover time series81 for each year from 1992 to 2018. The data was available for 98% of analysed sites and for 99% of sites. We computed the entire upstream catchment for each point occurrence using the r.water.outlet module and calculated the percentage cover of each land cover category within this area. The areas of cropland and urban land were calculated by dividing the percentage of upstream area averaged across the years at each site.
We extracted monthly climatic predictors from the TerraClimate dataset79 for 1967–2020, which covered all sites and years. For each site, we identified the sampling month and computed the mean monthly climatic value for the corresponding subcatchment. Climatic predictors were calculated for the 12 month period preceding the average temperature for each site. The value of trend in precipitation and temperature over the period was calculated using the R package brms 80. The models were like the models used to calculate site-level biodiversity metric trends, which had a trend estimated as the coefficient of a continuous year effect. The TerraClimate dataset is associated with uncertainties in areas of complex terrain, but our large number of sampling sites, relatively good station coverage and the low physiographical complexity of most site locations should have minimized error in our analyses.
We used the MERIT hydro DEM76) to calculate stream topographical and topological predictors. We computed the average elevation (m), elevation difference between the site and the upstream sub catchment and slope for each sub catchment using the r.univar module. To create a proxy for dam impacts, we calculated the network distance between each site and each upstream dam using the Global Reservoir and Dam Database (v.1.3)78. For dam impact score calculations, see Supplementary equation (1).
Source: The recovery of European freshwater biodiversity has come to a halt
Taxonomic aggregation of European invertebrate taxons. II. Identification of 61 non-native species
In total, we identified 61 non-native species. The initial analysis of native and non-native species was restricted to the 1,299 sites at which taxa were identified to species or a mixed taxonomic resolution; we excluded the remaining 517 sites due to the coarse (primarily family level) taxonomic resolution, which does not allow for reliable identification of non-native species. There are only 898 sites where non-native species were recorded, and estimates of the trends in richness and abundance were limited to them. The two most abundant non-native species were the New Zealand mud snail, Potamopyrgus antipodarum (≥1 individual present in ≥1 year at 81% of sites) and the North American bladder snail, Physella acuta (34% of sites).
We took the following steps to fill in gaps due to missing trait data. First, when trait data were not available at the original identification level (15.9% trait coverage across taxa), we used genus-level trait data, resulting in 48.2% coverage. Genus-level trait data can be used to represent the most interspecific variation among freshwater invertebrates. When the trait data for taxa were not available for identification, we replaced missing values in trait modalities with the median of trait profiles of all species from the full list of taxons. The median value of trait profiles of all genera within a family was replaced by missing values in trait models for taxa identified to family level. The lack of accurate phylogenies for many invertebrate taxa, low trait coverage at the species level and mixed taxonomic resolution across sampling sites prevented the use of other gap-filling approaches, but taxonomic aggregation generally aligns well with expert trait assignments65.
Our compiled time series represent different stream types and stream orders from a large geographical area of Europe. Detailed information about the purpose for which the data was collected is unavailable for some time series. These data were not selected randomly but were collected from available studies that met our six criteria. As these sites were exposed to different levels of anthropogenic impacts, we can’t rule out biases that arise from differing representation of sites from different impact levels.
All sampling years from 13 to 518, between 13 and 516 taxa were taken. A majority of the sites were identified to their species, while a few sites were mixed in status and a few had only family in them. 2,724 taxa from 959 genera were recorded. We list the time-series locations, durations and characteristics in Supplementary Table 2 and then we list the number of sites in Supplementary Table 3.
We ran linear mixed-effects models to synthesize site-level data and estimate overall mean trends. The models included site-level trend estimates as the response, an overall intercept and two random effects (country and study identity) as predictors. The uncertainty of the estimates of the mean trend was represented by brms 80, used to estimate the site-level trends.
Recent Posts
- investigators say that 2 were killed and six were injured in Florida state shooting
- Stem-cell therapies should not be rushed
- A trial is currently underway of iPS-cell derived dopaminergic cells
- The first meeting of CDC vaccine advisers is still going strong
- The genetic inheritance that could change the treatment of Alzheimer’s