In low-resource settings there are causes and consequences of child growth faltering
by admin
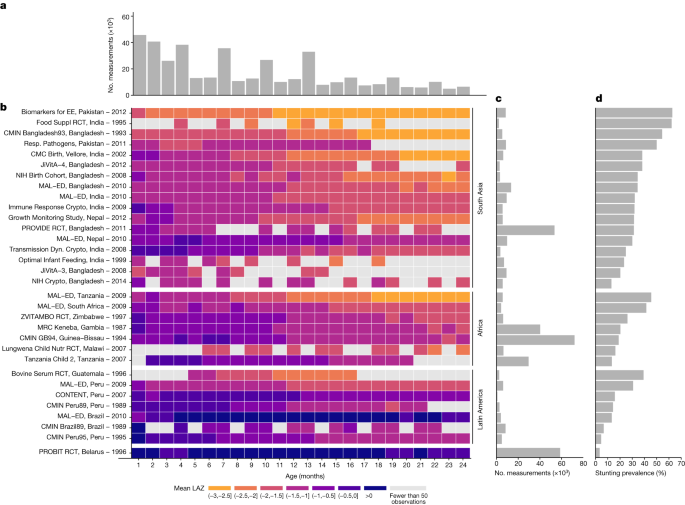
Mapping the Quality of Individual Cohort Datasets for the Development of Child-Aged Adults. I. Statistical Methodology and Analytical Methods
The Ki data team assessed the quality of individual cohort datasets by checking the range of each variable for outliers and values that were not consistent with expectation. z-scores were calculated using the median of replicate measurements and the 2006 WHO child growth standards30. In a small number of cases, a child had two anthropometry records at the same age, in which case we used the mean of the records. Analysts looked for expected correlations in the scatter plots, for example, length by height, age, or weight. Once the individual cohort data were mapped, analysts conducted an internal peer review of published articles for completeness and accuracy. Analysts contacted contributing investigators to seek clarification about potentially erroneous values in the data and revised the data as needed.
We stratified the above outcomes within the following subgroups: child age, grouped into one- or three-month intervals (depending on the analysis); the region of the world (Asia, sub-Saharan Africa, Latin America); sex of child; and the combinations of those categories. We obtained country-level data on the percentage of gross domestic product devoted to healthcare goods and spending from the United Nations Development Programme57 and the percentage of the country living on less than US$1.90 per day and under-5 mortality rates from the World Bank58. In years without available data, we linearly interpolated values from the nearest years with available data and extrapolated values within 5 years of available data using linear regression models based on all available years of data. We also considered the additional subgroup of gross domestic product, gender development index57, gender inequality index57, and the Gini coefficients. There was no way to separate the effects of each variable due to their strong correlation with geographic region. Thus, we did not conduct subgroup analyses for these variables.
There were analyses that pooled results across the study. We estimated each age-specific mean using a separate estimation and pooling step. We first estimated the mean in each cohort, and then pooled age-specific means across cohorts, while allowing for a cohort-level random effect. This approach enabled us to include the most information possible for each age-specific mean, while accommodating slightly different measurement schedules across the cohorts. LAZ is the stunting incidence estimate for which each cohort contributed data.
We used maximum-lihood estimation to calculate the mean, standard deviation, and skewness of the LAZ distributions. We fitted models separately by cohort.
Random-effects models assume that the true population outcomes θ are normally distributed (θ ~ N(μ, τ2)), in which N indicates a normal distribution and θ has mean μ and variance τ2. To estimate outcomes in this study, the random-effects model is defined as follows for each study in the set of i = 1, …, k studies:
in which ({\bar{\theta }}_{w}) is the weighted mean outcome in the set of k included studies, and wi is a study-specific weight, defined as the inverse of the study-specific sampling variance vi. θi is the estimate from study i.
Both outcomes were pooled on the logit scale to constrain confidence intervals between 0 and 1. Although the probit transformation more closely resembles common distributions for physiologic variables, in practice the logit transformation produces nearly identical estimates and is more convenient for estimation. For cohort-stratified analyses, which did not pool across studies, we estimated 95% confidence intervals using the normal approximation (Supplementary Note 7).
To examine the distribution of LAZ among children with stunting reversal, we created subgroups of children who experienced stunting reversal at ages 3, 6, 9 and 12 months and then summarized the distribution of the children’s LAZ at ages 6, 9, 12 and 15 months. The mean difference between the LAZ at older ages compared to the stunting reversal at the younger ages was estimated within each age interval. Pooled analyses used random-effects models for the primary analysis and fixed-effects models for sensitivity analyses as described above.
If all of the population’s exposure was set at an ideal low-risk reference level, the PAF is a proportional reduction in cumulative incidence. We estimated stunting and wasting from birth to 6 months, from 6 to 24 months, and from 6 to 24 months. For each exposure, we chose the reference level as the category with the lowest risk of stunting or wasting.
The prevalence ratios are compared to the reference level at birth, 6 months, and 24 months. Prevalence was estimated using anthropometry measurements closest to the age of interest and within one month of the age of interest, except for prevalence at birth which only included measures taken on the day of birth.
Cumulative incidence ratios (CIRs) between comparison levels of the exposure, compared to the reference level, for the incident onset of outcomes between birth and 24 months, 6 and 24 months, and birth and 6 months.
Optimal individualized intervention impact. The effect of an individualized intervention on an exposure63 was estimated using a variable importance measure methodology. In order to determine the optimal intervention, an individualized rule for the lowest-risk level of exposure was created based on the covariates. The covariates used to estimate the low-risk level are the same as those used to adjust for it. If every child is exposed to the optimal level, the effect of the individualized intervention will be derived from the variable importance measure. The reference level could vary across participants because we didn’t specify it in the PIE and PAF parameters.
To adjust for potential confounders and reduce the risk of model misspecification, we use a two-stage estimation strategy that incorporates machine learning algorithms and still provides valid statistical information. The effects of covariate adjustment on estimates compared to unadjusted estimates is shown. Cross-validation is a method used to choose a weighted combination of predictions in a machine learning method. We included in the library, simple means and generalized linear models. The super learner was fit to maximize the tenfold cross-validated area under the receiver operator curve (AUC) for binomial outcomes, and minimize the tenfold cross-validated mean-squared error (MSE) for continuous outcomes. That is, the super learner was fit using nine-tenths of the data, while the AUC/MSE was calculated on the remaining one-tenth of the data. Each fold of the data was held out in turn and the cross-validated performance measure was calculated as the average of the performance measures across the ten folds. This approach is robust in finite sample since it uses unseen sample data to measure the estimators performance. Also, the super learner is asymptotically optimal in the sense that it is guaranteed to outperform the best possible algorithm included in the library as sample size grows. The first estimate obtained through super learner is updated to yield the second one. We fit models without the learning step and within each cohort to measure the exposures, so we can estimate the R2 of models. We then pooled cohort-specific R2 estimates using fixed-effects models.
We looked covariate missingness by study and evaluated the effect of covariate missingness by comparing results with median/mode missingness imputation in a complete case analysis. We compared estimates pooled using random-effects models, which are more conservative in the presence of heterogeneity across studies, with estimates pooled using fixed effects (Supplementary Note 3), and we compared adjusted estimates with estimates unadjusted for potential confounders (Supplementary Note 4). All exposure levels were plotted for child growth trajectory in the Supplementary Note 5. The PROBIT trial was dropped due to re-estimation of the attributable differences of exposures at 24 months. Point estimates and confidence intervals from all age, exposure and growth outcome combinations (as presented in Extended Data Fig. 2) are plotted in Supplementary Note 7.
We estimated influence curve-based, clustered standard errors to account for repeated measures in the analyses of recovery from wasting or progression to severe wasting. We assumed that the children were the independent units of analysis unless the original study had a clustered design, in which case the unit of independence in the original study were used as the unit of clustering. We used clusters as the unit of independence for the iLiNS-Zinc, Jivita-3, Jivita-4, Probit, and SAS Complementary Feeding trials. We used the normal approximation to estimate the confidence intervals.
We did not estimate relative risks between a higher level of exposure and the reference group if there were 5 or fewer cases in either stratum. If the reference strata were not sparse, we still estimated the relative risks between exposure and reference. We only included a covariate for every 10 observations if there was a sparse combination of the exposure and outcome.
Outcome ,95 %,rmconfidence,rmInterval.
For PAFs of exposures on the cumulative incidence of wasting and stunting, the pooled cumulative incidence was substituted for the outcome prevalence in the above equations. PIEs are unbounded with symmetrical confidence intervals so we used this method instead of pooling PAFs.
For Fig. 4a,b, mean trajectories estimated using cubic splines in individual studies and then curves were pooled using random effects62. Curves estimated from all anthropometry measurements of children taken from birth to 24 months of age within studies that measured the measure of maternal anthropometry.
We first estimated the mean in each cohort, and then pooled age-specific means across cohorts, while allowing for a cohort-level random effect. The mean difference between the LAZ at older ages compared to the stunting reversal at the younger ages was estimated within each age interval. The effect of an individualized intervention on an exposure was estimated using a variable importance measure methodology.
Recent Posts
- investigators say that 2 were killed and six were injured in Florida state shooting
- Stem-cell therapies should not be rushed
- A trial is currently underway of iPS-cell derived dopaminergic cells
- The first meeting of CDC vaccine advisers is still going strong
- The genetic inheritance that could change the treatment of Alzheimer’s