How a piece of technology could be used to improve treatments
by admin
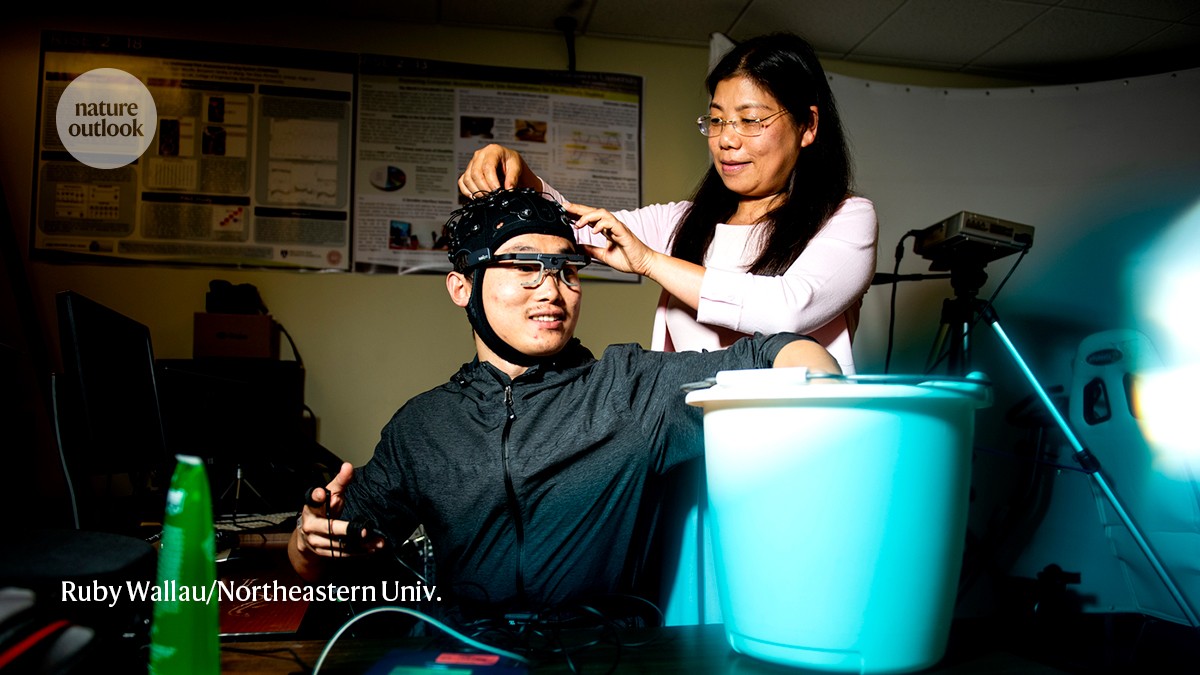
Pain Detection with Biological Outputs: A New Laboratory to Detect and Predict Complications of Neurological Paradigms
“You have to give each drug at least six weeks to see if it works, before trying the next one,” Russo says. Whether or not it eases the pain, a drug can cause side effects. After cycling through each migraine drug individually, the team started testing drug combinations. She got control of her headaches after the 24th medication trial.
It will take a lot of resources and manpower to build the large data sets that are necessary to reach this point. But, prescribing an effective pain treatment from the start for each person is likely to be a much more cost-effective investment than chasing new pain drugs, Russo argues. “You are never going to get that by having the world’s best super-duper drug, because there’s too much heterogeneity in human pain for that to be ever possible,” he says. “But it may be possible to predictively say, of these 12 good existing drugs, I can now reject 11 of them and select the one that will work for the patient in front of me.”
At the laboratory I visited at Northeastern University in Boston, Massachusetts, mechanical engineer Yingzi Lin and her colleagues at are collecting a wide range of physiological parameters. As well as information of the kind presented by the sensor that I wore, they are gathering eye-tracking and electrical-brain-activity measurements to identify a reliable signature of pain. The task is to extract meaningful patterns from this jumble of biological outputs, each of which is indicative, in its own way, of the pain experience. “It’s a lot of data,” Lin says. It is a challenging problem.
An optimistic person who breaks their arm mountain biking on a weekend away with friends to celebrate a promotion could experience pain differently to a pessimistic person who breaks an arm cycling from their monotonous job to their home, on which they are behind on the mortgage repayments. Depression and anxiety can be risk factors for chronic pain. “Nobody experiencing chronic pain is in the same place at the same time,” she says. Everything else around them, including what they have experienced before and what they are anticipating, is a part of the pain.
Pain phenotyping using machine learning and artificial intelligence: a case study in European paincare biopain management systems (PainCare Biopaincare)
Washed out to the human eye, at least. “When we compared the EEGs of people with pain versus those with no pain, standard statistical analysis showed no difference,” Saab says. When Saab and his collaborators trialled a basic form of machine learning to process the data, however, the artificial intelligence (AI) algorithm could detect something. In between people with chronic lower back pain and people with healthy controls, but not a stimulator, the algorithm was able to separate them from each other on the basis of their pain. The algorithm had an of accuracy almost 80%, Saab says, showing that even a simple AI can outperform conventional statistics for the task. “Basic machine learning is picking up signals that classic methods and the human eye are not capable of detecting.”
Vollert has seen similar results from applying basic machine learning to an approach called sensory phenotyping. He has used this technique with people experiencing neuropathic pain, which arises from nerve damage. The aim is to understand the changes to the sensory system that accompany pain. “With a strong headache, for example, many people get very sensitized to sounds or smells,” says Vollert.
The early results were promising. When I first published my work on structural brain scans to classify the presence or absence of lower back pain, we got 76% accuracy. Progress then stalled. He says that since then, the accuracy hasn’t improved.
By pooling data from multiple biomarker techniques, however, a stronger signal should emerge. “Where the field is going is to bring all these data sets together,” says Vollert, who is currently participating in two such efforts in Europe: the IMI-PainCare Biopain consortium led by Rolf-Detlef Treede, a neuroscientist at the University of Heidelberg in Mannheim, Germany, and Painstorm, led by David Bennett, a neurologist at the University of Oxford, UK. “To build really good models, we need prospective data sets that collect all kinds of marker information in parallel from the same patients,” Vollert says. That data set is what we are generating at the moment.
With multimodal data in hand, advanced AI tools that are more powerful than the simple machine-learning models previously deployed will be crucial to categorizing people into subgroups and treatment selection, says Vollert. He says they’re looking for models that go much deeper into the rich data sets.
The latest advanced AI models — including ChatGPT and the AlphaFold protein folding prediction tool — use a machine-learning approach called deep learning neural networks. The models start making connections between the points on the input data set to generate their output. The goal is that if deep learning neural networks are combined with big new data sets, then accuracies can be significantly higher.
One caveat is that these advanced AI techniques apply non-interpretable models to biomarker data analysis. In his early work with machine learning, I could tell you if the brain regions were contributing to the model. “With the new and improved versions, we can get incredible accuracy, but I can’t tell you what’s driving it.”
There is a problem for pain-medicine practitioners with non-interpretable modelling. As clinicians, we want to understand the basis of a medical recommendation and not blindly take the word of a machine if it’s not right.
It took three years for her to find a solution to her headaches. There are lots of alternatives to treat people with chronic migraines, which is not the problem. Finding the right treatment for chronic pain sufferers is a very hard game to play, testing treatments one by one in the hopes of finding a match.
Her headaches would hit on a daily basis. The doctor who treated her at Hunter Pain Specialists says she vomited and had to lie down between patient consults. “Then she’d have to get up, rinse her mouth and see the next patient.”
Pain Management Using Artificial Intelligence: a Case Study of a Backache Patient in a Cold-exposure Task using a Sensing Device
“Hmm, it’s not really working,” says Lin, acknowledging that much optimization work remains. “I think maybe we should stop.” As I withdraw my hand from the icy cold, the pain quickly subsides — and I don’t need an objective measure to tell me when, after a few moments, the pain is gone.
The platform proved glitchy. The pain scores on the app trended upwards when I submerged my hand in ice water. The readings were inconsistent, however, fluctuating as I experienced a steadily increasing sensation of pain.
When I tried the cold-exposure task in her lab, I had only one sensor on my body feeding data into COMPASS. Facial tracking, measurements of pupil diameter and monitoring of brain activity weren’t recorded.
In a study of pain, the researchers found that a model using only facial expressions and brain activity signals was more effective than other approaches, like a more limited subset of indicators. There is a front. The journal Neurosci. 16, 833571, is available.
Lin is using data from people with chronic low back pain to fine-tune her machine-learning algorithms for her platform. The artificial-intelligence tools have proved to be far superior to the capabilities of single clinicians, for example, by being able to extract valuable insights from diverse data sets.
There is a snapshot of a person’s pain at a point in time. That’s fine for some situations, such as an assessment by a physician, but it can’t track fluctuations in pain levels over extended periods.
But the cost and complexity of brain-recording technologies make these methods unsuitable for clinical use. “It has to be practical and portable,” Saab says — which is where simpler diagnostic tools and wearable devices come in.
The platform can only be used for surgery with people who cannot communicate their pain levels and who are under anaesthesia. By allowing clinicians to adjust painkiller dosages in line with an individual’s pain, it aims to optimize pain management and mitigate the risks associated with unnecessary opioid prescriptions.
Moreover, many individuals — including babies, people who are non-verbal, people who are critically injured and those under sedation — cannot communicate their symptoms effectively. This makes it difficult to manage and treat pain, and complicates the delivery of analgesia.
As discomfort escalates to pain, a sensor strapped to my chest detects changes in my heart rate, breathing pattern, skin conductance and other bodily responses. The pain score is created by the signals that have been processed. Displayed on a smartphone app, my pain level is 4.
A graduate student has his hand on my arm. The sensation of pressure on a patch of skin that’s roughly the size of a small coin starts to hurt, even though it doesn’t seem like much.
A study conducted by researchers in Germany found that a model using only facial expressions and brain activity signals was more effective than other approaches, like a more limited subset of indicators. It found that a model using only facial expressions and brain activity signals was more effective than other approaches, like a more limited subset of indicators.