Your blood is indicative of how your organs are aging
by admin
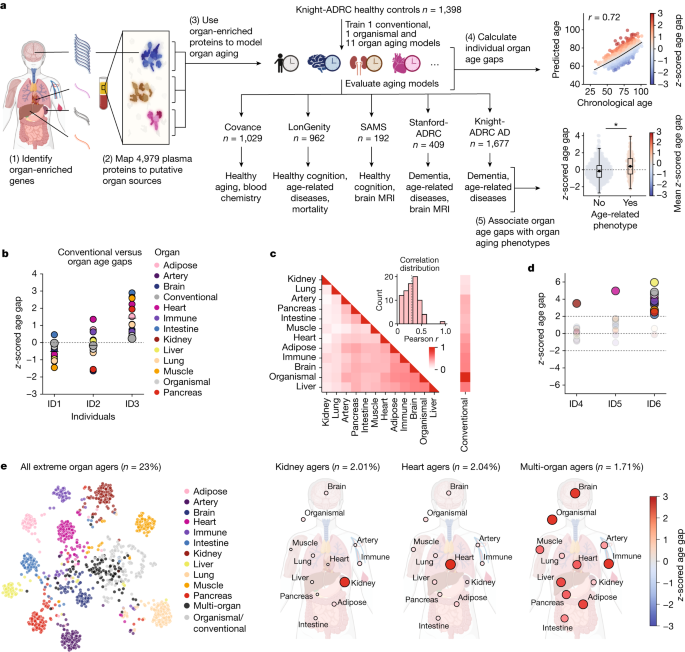
A longitudinal observational study of cognitive, neuropsychological, imaging and biomarker assessments for clinical dementia subjects and age-matched controls at the Knight-ADRC cohort
The Knight-ADRC cohort is an NIA-funded longitudinal observational study of clinical dementia subjects and age-matched controls. Research participants at the Knight-ADRC undergo longitudinal cognitive, neuropsychologic, imaging and biomarker assessments including Clinical Dementia Rating (CDR). Among individuals with CSF and plasma data, AD cases corresponded to those with a diagnosis of dementia of the Alzheimer’s type (DAT) using criteria equivalent to the National Institute of Neurological and Communication Disorders and Stroke-Alzheimer’s Disease and Related Disorders Association for probable AD58, and AD severity was determined using the Clinical Dementia Rating (CDR)59 at the time of lumbar puncture (for CSF samples) or blood draw (for plasma samples). Controls received the same assessment as the cases but were nondemented (CDR = 0). Blood samples were collected in the vacutainer purple top and immediately taken to a lab for further analysis and storage at an appropriate temperature. The plasma was stored in monitored −80 °C freezer until it was pulled and sent to Somalogic for data generation. The research was approved by the Institutional Review Board of the Washington University School of Medicine. There were three thousand participants included in the present study. Cohort demographics and clinical diagnostic categories are summarized in Supplementary Table 1.
Source: Organ aging signatures in the plasma proteome track health and disease
Using the SomaScan Data Set to Identify Organ-Enriched Genes, Plasma Proteins and Gene Tissue Expression Atlas (GTEx)
Over 700 publications have been made with the analysis of 1 million samples at the time of this publication. There is minimal replicate sample variability64,65 (coefficient of variation, CV). A subset of the SomaScan samples have been successfully tested as laboratory-developed tests, and delivered to patients in the context of medical management.
Standard Somalogic normalization, calibration and quality control were performed on all samples54,61,62,63. Briefly, pooled reference standards and buffer standards are included on each plate to control for batch effects during assay quantification. The samples are normalized within and across the plates using the median signal intensities specified in the reference standards. An adaptive maximum likelihood procedure was used to normalized the samples. If the signal intensities deviated significantly from the expected range, samples are excluded from analysis. raw data is the data provided from the company, which is considered the resulting expression values.
Direct comparisons across two v.4 and three v.4.1 cohort were made possible with the application of the v.4 v.4.1 multiplication scaling factors. The correlation between both v. 4 and v.4.1 and low estimated replicate coefficients of variation64 were discarded. There were 4,778 involved in the analysis. The raw data were log10 transformed before analysis, as the assay has an expected log-normal distribution.
Approximately 900 probes measuring mostly high and mid abundance proteins, have been confirmed with either data dependent acquisition or multiple reaction monitoring mass spectrometry due to sensitivity limitations.
2,860 probe measurements are associated with genetic variation in the cis- protein quantitative trait loci.
Roughly 1,150 probes are unable to detect the signal from the closest neighbor that is most closely related to the cognate one.
We used the Gene Tissue Expression Atlas (GTEx) human tissue bulk RNA-seq database18 to identify organ-enriched genes and plasma proteins (Extended Data Fig. 1). Tissue gene expression data were normalized using the DESeq2 (ref. 67) R package. We define organ-enriched genes in accordance with the definition proposed by the Human Protein Atlas19: a gene is enriched if it is expressed at least four times higher in a single organ compared to any other organ. Within GTEx, we grouped tissues of the same organ together, such that a gene’s expression level for a given organ was the maximum gene expression value among its subtissues. For example, all GTEx brain regions were considered subtissues of the brain organ. We define the immune organ, which is not a GTEx tissue, as expression in the blood and the spleen tissues. The genes were mapped in a manner that allowed us to determine the amount of the 4,979proteins that were quantified.
We performed the steps for each aging model to calculate the sample age gap. We fit a local regression between predicted and chronological age using the lowess function from the statsmodels69 python package with fraction parameter set to 2/3 to estimate the true population mean (Supplementary Fig. 3e). Local regression is used in place of linear regression because of the evidence that the plasma proteome changes with age1, which has been found in all five cohort studies. There are 8. Individual sample age gaps were then calculated as the difference between predicted age and the lowess regression estimate of the population mean. The age gaps were calculated according to the different cohort differences. Age gaps were z-scored per aging model to account for the differences in model variability (Supplementary Fig. 3f). This allowed for direct comparison between organ age gaps in downstream analyses.
We used the published coefficients14 to calculate the phenotypic age of participants in the Covance cohort using albumin, creatinine, glucose, c-reactive protein, % lymphocyte, mean cell volume, red cell distribution width, alkaline phosphatase, white blood cell count and age.
The Cox proportional hazard models were used to calculate an association between organ age gaps and future risks of death and heart failure, and increase in dementia rating. Models were tested using the lifelines71 python package. Kaplan Meyer curves were generated at population-average covariate values in the relevant subject populations.
The inverse variance weighted fixed effect model was used for the meta-analyses which compared and aggregate effect sizes and confidence intervals from several cohorts.
Applying FIBA to understand the association with cognition in organ aging models: The CDR-Global score from the Knight-ADRC cohort
FIBA is an adaptation of permutation feature importance (PFI)72 (Extended Data Fig. 6a). PFI is traditionally used in machine learning to assess how much a model depends on a given feature for prediction accuracy of the target variable. When value from a single feature are randomized, the decrease in a model’s performance is known as the PFI score. The original prediction accuracy would be the difference between the model and the prediction accuracy after randomization of a single feature. The final PFI score is the mean PFI score from five randomizations.
We applied FIBA to understand aging model protein contributions to associations with cognition using the CDR-Global score. The mean FIBA score after five permutations was calculated for all 500 bootstraps for all organ aging models (Supplementary Table 15). A protein was defined as significant (FIBA+) if less than 5% (empirical single-tailed P < 0.05) of its FIBA scores across bootstraps was negative. Only those with nonzero coefficients in a certain number of bootstraps were considered. FIBA+ organ-specific proteins were used to train new cognition-optimized aging models from cognitively unimpaired individuals in the Knight-ADRC cohort.
Biological pathway enrichment analyses were performed using g:Profiler73 with the all human genes set as the background distribution. Protein–protein interaction networks were generated using the STRING database74.
Source: Organ aging signatures in the plasma proteome track health and disease
Brain Age Estimation from Whole-Brain MRI Scans using FreeSurfer, a Pretrained Model and Genome Analysis Toolkit
Whole-brain MRI scans were collected from all subjects in the Stanford-ADRC and SAMS cohorts. All MRI data was collected at the Stanford Richard M. Lucas Center for Imaging. There were 271 people who had their brains scanned on the GE Discovery MR750. Grey matter volumes were determined by using T1-weighted SPGR scans and flip angle of 9. A total of 134 subjects underwent MRI scanning on a hybrid PET/MRI scanner (Signa 3 tesla, GE Healthcare). T1-weighted SPGR scan were collected (TR/TE/TI = 7.7/3.1/400 ms, flip angle = 11, 1.2 × 1.1 × 1.1 mm) and used to define grey matter volumes.
The Free Surfer75 softwarepackage was used to implementROI labelling. In brief, structural images were bias field corrected, intensity normalized and skull stripped using a watershed algorithm. These images underwent a white matter-based segmentation, grey/white matter and pial surfaces were defined, and topology correction was applied to these reconstructed surfaces. Each subject had a subcortical and cortical ROI in their native space, in FreeSurfer.
The brains of 541 samples in SAMS andSTANFORD-ADRC were matched with the brain’s proteomic data.
There is a pretrained scheme called BARACUS that we used. 76) to estimate brain age from FreeSurfer v.5.3 processed T1-w scans. The difference between the cortical thickness and surface area values were used as input to the machine model. 566 women with no objective cognitive impairment were trained on the model. It returns an estimate of brain age for this method with its results.
AD polygenic risk scores (PRS) were calculated in the Stanford-ADRC cohort to compare to the CognitionBrain age gap. PRSs were determined from whole-genome sequencing. The Genome Analysis Toolkit workflow High-confidence variant calls were made using Germline short variant discovery and the reference genome. Six individuals were excluded from further whole-genome sequencing analysis due to discordance between their reported sex and genetic sex. APOE genotype (ε2/ ε3/ ε4) was determined using allelic combinations of single nucleotide variants rs7412 and rs429358. The largest AD GWAS so far identified independent genes that were used to compute AD PRS. Namely, the 84 variants and their effect size available from Tables 1 and 2 in ref. 30 were utilized, in addition to rs7272 and rs429358. The data from the individual genos and the list of variant with their effect size as input were used to formally compute the PRS. Three individuals with pathogenic mutations PSEN1 or GBA were removed from this analysis.
Source: Organ aging signatures in the plasma proteome track health and disease
Lifetime versus Age: A Multi-Site Study in the U.S. for Pedestrians with Multiple Diseases and Demographic Factors
The details of the study were published before. Covance is a multi-site study that looked at lifespan at five US hospital sites in 2008. There were 1,028 subjects included in the analyses. The demographic characteristics are summarized in Supplementary Table 1. Exclusion criteria for the study include hypertension, self- reported treatment for a cancer other than the skin in the last two years, pregnancies, autoimmune condition or other inflammatory condition, self- reported chronic glomerularitis, or chronic bronchitis.
Clinical blood chemistry was performed on the same samples, including a complete blood count and comprehensive metabolic panel, lipid panel and liver function tests. Basic physical workup (blood pressure, pulse and respirations) was also collected. All participants were asked to fill a survey about their lifestyles, which included what they smoke, how much they drink, and their intake of different meats and vegetables.
The subjects in the LonGenity cohort had to take a cognitive exam. The relative performance of the subject in the Free and Cued Selective Reminding Test were used to determine the Overall Cognition score. For each task a standardized score (z) was calculated based on the population. The combined score for each task was then used to create the aggregate score.
As people age, their cells undergo a raft of biochemical changes that lead to organ damage and, eventually, death. A study shows that organs can age at different rates in different people and that the age of a given organ is related to chronological age.
The Knight-ADRC cohort is an NIA-funded study of clinical dementia subjects and age-matched controls. Among individuals with cerebrospinal fluid (CSF) and plasma data, Alzheimer’s (AD) cases corresponded to those with a diagnosis of dementia of the Alzheimer’s type using criteria equivalent to the National Institute of Neurological and Communication Disorders and Stroke- Alzheimer’s Disease and Related Disorders Association for probable AD58.
Recent Posts
- David Souter died at the age of 85
- There is continuity in the United States Southwest based on the oral history and genetics of Picuris Pueblo
- There is a widespread power interruption in Spain and neighboring countries
- Donald Trump signed an executive order toexpedite deep sea mining
- For a Times reporter who covered him, Francis was always a surprise