The UK Biobank has rare variant associations with plasma protein levels
by admin
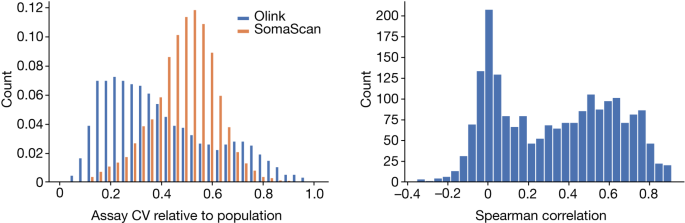
Recursive Conditional Analysis of Human GWAS Catalogue Variants in High LD with Sentinel pQTLs
We identified all variants reported in the NHGRI-EBI catalogue of human GWAS74 (excluding proteomics studies) in high LD (r2 > 0.8) with sentinel pQTLs based on Olink-UKB-BI data and Icelandic SomaScan data (Supplementary Tables 38 and 39). For each sentinel pQTL association, we also identified a 95% credible set of variants (variants that most parsimoniously explain regional association75) likely to include the causal variant76. We then checked whether GWAS catalogue variants in high LD with the pQTL variant (with r2 > 0.8 with the pQTL) were included in the credible set. In addition to high LD between the disease-associated variant and both the pQTL and a variant in the credible set, for the highlighted examples, we estimated the posterior probability of statistical colocalization for the variants associating with disease and protein levels when they were not identical and when we had access to the necessary statistics77.
We performed recursive conditional analysis separately for each assay and each chromosome based on individual-level genotypes. The candidate set of sequence variants we restricted this analysis to have a P 5 106. If the variant, v1, with the lowest P value had P <1.8 × 10−9, we removed v1 from the candidate set and the association of all other variants in the candidate set was recomputed, conditional on v1. If any variant in the candidate set had P < 1.8 × 10−9, we assigned the label v2 to the variant with the lowest P value, removed v2 from the candidate set, and calculated the conditional association of the variants remaining in the candidate set given v1 and v2. We repeated this process until no variant in the candidate set had P < 1.8 × 10−9. Conditional analysis for two assays did not finish for all secondary signals but did return values for sentinel pQTLs.
The PEACOK R package implementation is intended to separate the generation of matrix from the testing. It allows statistical tests to be seperated from each other on different computing environments. Various downstream analyses and summarizations were performed using R v.3.6.1 (https://cran.r-project.org). R libraries data.table (v.1.12.8; https://CRAN.R-project.org/package=data.table), MASS (7.3-51.6; https://www.stats.ox.ac.uk/pub/MASS4/), tidyr (1.1.0; https://CRAN.R-project.org/package=tidyr) and dplyr (1.0.0; https://CRAN.R-project.org/package=dplyr) were also used.
For UKB tree fields, such as the International Classification of Diseases tenth edition (ICD-10) hospital admissions (field 41202), we studied each leaf individually and studied each subsequent higher-level grouping up to the ICD-10 root chapter as separate phenotypic entities. The controls were restricted for the tree-related fields because we did not want participants to be contaminated with diseases caused by genetically related diagnoses. A minimum of 30 cases were required to be studied. In addition to studying UKB algorithmically defined outcomes, we studied union phenotypes for each ICD-10 phenotype. The union phenotypes are marked by a certain prefix and are available in Table 1 of Wang et al.11.
To detect putative CH somatic variants, we used the same GRCh38 genome reference aligned reads as for germline variant calling, and ran somatic variant calling with GATK’s Mutect2 (v.4.2.2.0)67. The 74 genes that were selected were used for the analysis as being recurrently Mutational in myeloid cancers. To remove potential recurrent artifacts, we filtered variants using a panel of normals created from 200 of the youngest UKB participants without a haematologic malignancy diagnosis. Priors were generated with LearnRead Orientation Model and used to perform subsequent filtering with GATK.
From variant calls, it was possible to identify somatic anomalies using a list of variant effects and specific missense variations (Supplementary Table 20). Only PASS variant calls with a 0.04 variant allele Frequency and an allelic depth greater than or equal to 3 were included. We found all of the identified variant for each gene and limited further analysis to a set of 15 genes.
An ExWAS reappraisal for the UKB cohort: analysis, validation, and quality control in a large sample of UKB exomes
The VAF cut-offs were considered for the collapsing analysis. We excluded 359 individuals diagnosed with a haematological malignancy predating sample collection and included body mass index (BMI) and pack years of smoking as additional covariates. Most of the significant (P ≤ 1 × 10−8) associations arose with a VAF ≥ 10% cut-off (Supplementary Table 13).
The UKB exomes cohort that was adopted for this refreshed PheWAS analysis was sampled from the available 469,809 UKB exome sequences. We excluded from analyses 118 (0.025%) sequences that achieved a VerifyBAMID freemix (contamination) level of 4% or higher68, and an additional five sequences (0.001%) where less than 94.5% of the CCDS (release 22) achieved a minimum of tenfold read depth69.
As an additional quality control check, we assessed the concordance of suggestive and significant ExWAS cis-CDS pQTLs (P < 1 × 10−4) corresponding to proteins that were measured in multiple Olink panels (CXCL8, TNF, IDO1 and LMOD1). Encouragingly, there was complete concordance across panels (Supplementary Table 18). We did not see any cis-CDS pQTLs with a P 1104 for IL-6) and SCRIB, but they were measured on multiple panels. Ideally, potential epitope effects could be assessed by testing whether cis-CDS pQTLs preferentially overlap with known binding sites for the antibodies used on the Olink platform. These data were unavailable on request.
The UKB samples were measured in Sweden. All samples were randomized and plated by the UK Biobank laboratory team prior to delivery. Samples were processed across three NovaSeq 6000 Sequencing Systems. Quality control measures at Olink’s facility resulted in NPX values for each member of the group. Olink has a relativeprotein quantification unit called NPX.
The UKB is a prospective study of approximately 500,000 participants aged 40–69 years at recruitment. Between 2006 and 2010 participants were recruited in the United Kingdom. The average age at recruitment for sequenced individuals was 56.5 yr and 54% of the sequenced cohort comprises those of the female sex. Health records that are periodically updated by the UKB are one of the many data points included in Participant data. All study participants provided informed consent.
The UKB Ethics Advisory Committee is in charge of the protocols for the UKB.
Samples likely to be incorrectly labelled were identified based on individual predictions of sex by protein levels, and of protein levels by genotypes. Whole plates or individual rows or columns of samples, identified as being majority likely incorrectly labelled, were excluded from the UKB-PPP data. There were 13 whole plates, five rows or columns of samples, which were not included in the total 1,179 samples. From the 1536 set of assays, this resulted in the exclusion of four whole plates and seven rows or columns of samples, in total 404 samples. Furthermore, in the 1536 set of assays, a single panel was excluded for two plates, affecting 174 samples.
Linear Regression was used to estimate the association of proteins levels and quantitative traits. Logistic regression was used to calculate the association ofProtein levels with a prior disease. The age and sex of the individual were adjusted to take into account the time that had passed since the collection.
Olink Explore: a comparison platform for the evaluation of the CV of repeated samples, SomaScan, and Uniprot, Olink, UniProt, and UProt
To be able to use the CV of repeated samples to compare the two platforms, we suggest considering the CV of repeated measurements relative to the expected CV of the assay if the repeated measurements were not of the same sample, but of samples selected at random from the population (Supplementary Fig. 1). This metric should range between 0 and 1 and is approximately equal to the ratio of the s.d. of repeated measurements to the s.d. of all measurements. The CV ratio of 0 is a sign that the measurements are completely correlated and that the precision is 100%. The CV ratio requires that the s.d. are the same as the random s.d. The platforms were evaluated using the ratio of the observed CV to the expected CV as a measure of their performance.
External data can be found in the GWAS catalogue, the GTEx project, and the HumanProtein Atlas.
We used the software in conjunction with the ones described above. The GATK resource bundle is the same as the BACMQC and GraphTyper. The genomics and pQTL processing pipelines have been extensively described previously2,12. Olink Explore is used to process data generated on the Olink platform. Data were analysed and figures generated using Python (version 3.9.1), along with packages numpy (version 1.20.3), scipy (version 1.7.1), matplotlib (version 3.4.3), and pandas (version 1.3.0), and R (version 3.6.0).
We identified the assays by using their UniProt IDs. 2,243 pairs of assays were used to target 1,848 UniProt IDs, 1, 86 Olink assays, and 1,994 Soma Scan assays (Supplementary Table 4).
The Olink measurements of the Icelandic plasma samples were performed at deCODE’s facility in accordance with the Olink Explore manual61. Quality control measures were the same as used by Olink for the UK Biobank samples.
Both Olink and SomaScan use dilutions of plasma samples to compensate for different concentrations of proteins in plasma13,59,62. Both of the platforms agree on placing the targeted set of proteins in the low, intermediate or high dilution groups.
A robust approach to genotyping and imputation in the whole genome of Icelanders: GraphTyper65 SNP variants with a median of 32
Illumina used a median of 32 for the whole genome of 63,118Icelanders. The variant were called by GraphTyper65. The data was used to impute genotypes when the samples were SNP genorated with Illumina chips. In total, 173,025 Icelanders were SNP genotyped, long-range phased and imputed based on the sequenced datasets. Where genotypes for an individual were missing for association studies, they were inferred using genealogic information if possible. The imputation learning set was based on the whole-genome of 15% of Icelanders which allowed variant imputation. In the past, genotyping and imputation have been described in greater detail. We restricted our analysis to variant numbers with 0.01% and imputation information less than 1 in order to produce 33.5 million variants. Other tools used for genotyping included bcl2fastq, Samtools, BWA, and the genome analysisTK lite.
Accuracy refers to how similar repeated measurements will be while CV is the s.d. of measurements divided by their mean. CV is not a good measure of accuracy in some cases. Indeed, if a platform were to produce random values in a tight range it would have a low CV but no accuracy.
We assumed that the two duplicate measurements were independent of each other, so we estimated the CV for the available measurements and compared it to the expectation of the CV. We used the robust median absolute deviation estimator to estimate the s.d. of the repeated measurements on the log-scale and inserted this estimate into the formula for the CV above, obtaining CVrep. If the CVrand is the same as the CV of all levels, then the ratio of s.d. to s.d. of the repeated measurements will be the same.
Both Olink and SomaScan use repeated measurements of control samples, specific to the platform, for quality control. When using two different samples on a single plate to evaluate CV, the evaluation doesn’t include the inter-plate variation if the samples are chosen randomly from the set of all samples. Comparing the CV ratio computed from the repeated control samples between the two platforms can therefore help comparison in terms of batch effects, with values closer to one suggesting that the platform is less susceptible to batch effects and closer to zero that the platform is more so (Supplementary Note 2, Supplementary Fig. 9).
Source: Large-scale plasma proteomics comparisons through genetics and disease associations
Large-scale plasma proteomics comparisons through genetics and disease associations II: A standardized mixed model with inflation, cryptic relatedness, and stratification72
We adjusted all of the measurement for sex, sample age, and age. We used a linear mixed model and standardized the residuals and the standardized values for genome-wide association testing. We used LD score regression to account for inflation in test statistics due to cryptic relatedness and stratification72.
We computed P values using a likelihood ratio test and adjusted for multiple testing by using the same significance threshold (1.8 × 10−9) as in our previous study on the Icelandic dataset2.
We tested whether the variant itself or the one in high LD affected the coding sequence of genes.
The number of variant tests in UKB is about five times larger than the number of test in Iceland. The difference isn’t due to normal things. However, the difference between them would result in a multiple testing correction threshold in UKB of 8.7 × 10−10 instead of 1.8 × 10−9. A total of 153 (1%) of the cis pQTLs are between those two thresholds and 1,608 (5%) of the trans pQTLs.
The threshold for replication between platforms was 0.05 and it needed to be in the same direction.
Source: Large-scale plasma proteomics comparisons through genetics and disease associations
Inverse cumulative distribution function for a given subcellular location: A study using the major histocompatibility complex of the human protein Atlas14
We considered sequence variants from the conditional analysis to belong to the same region if they were within 2 Mb of each other. We considered the major histocompatibility complex, which consists of 38 chr. 6:25.5-34.0MB) as a single region. We refer to the most significant variant in each region as the sentinel variant for the assay in the region, and other variants as secondary variants.
For a given P value threshold P, sample size N, effect size β, and MAF f, the probability of rejecting the null hypothesis of no association is given by 1 − F(X − 1(1 − P), 2Nβ2f(1 − f)), where X–1(·) denotes the inverse cumulative distribution function (inverse CDF) of the chi-squared distribution with one degree of freedom, while F(a, b) denotes the CDF of the non-central chi-squared distribution with one degree of freedom for quantile a and non-centrality parameter73 b.
Protein subcellular locations were determined using annotations from the Human Protein Atlas14, using the same approach as in Sun et al.6 where proteins annotated as ‘membrane’ by the Human Protein Atlas were considered to be membrane proteins, proteins annotated in the Human Protein Atlas as ‘secreted’ (but not ‘membrane’) considered to be secreted proteins, while other proteins were considered to be intracellular.
Blood was collected in tubes that were inverted 5 times and then taken to a hospital where it was put into a bin for 10 minutes. There were frozen samples at a temperature of 80C. During the thaw, Plasma aliquots were kept away from the light and kept on ice. Before measurement, the aliquots were mixed by inverting the tubes a couple of times and then centrifuged for 10 min at 3,220g at 4 °C.
Plasma samples were measured in duplicates with commercially available Simoa NF-light Advantage (SR-X) kit (Quanterix, cat. 103400). The samples were put into a solution of 4:1 and 25 l anti-NF lightImmunocapture beads and 20 l Biotinyylated detectors for 30 min. The bead-immunocomplexes were washed and resuspended before being placed in a culture dish of 100 l streptavidin and 800 rpm. After a second washing step, the bead-immunocomplexes and resorufin β-d-galactopyranoside were loaded onto an SR-X instrument (Quanterix) for processing and analysis.
We identified all variants reported in the NHGRI-EBI catalogue of human GWAS74 in high LD with sentinel pQTLs based on UKB-BI data and Icelandic SomaScan data. In addition to high LD between the disease-associated variant and both the pQTL and a variant in the credible set, we estimated the posterior probability of statistical colocalization for the variants associating with disease and protein levels when they were not identical.
Recent Posts
- investigators say that 2 were killed and six were injured in Florida state shooting
- Stem-cell therapies should not be rushed
- A trial is currently underway of iPS-cell derived dopaminergic cells
- The first meeting of CDC vaccine advisers is still going strong
- The genetic inheritance that could change the treatment of Alzheimer’s