Large-scale comparisons of genes and diseases
by admin
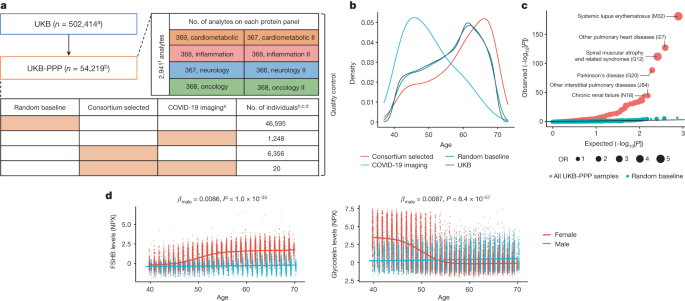
Quality control in the UKB-PPP data processing: genotype, exome sequencing, whole-body MMR, biological markers, physical and anthropometric measurements
Between 2006 and 2010 a large group of people aged 40–69 years were recruited into the UKB. Participant data include genome-wide genotyping, exome sequencing, whole-body magnetic resonance imaging, electronic health record linkage, blood and urine biomarkers, and physical and anthropometric measurements. Further details are available online (https://biobank.ndph.ox.ac.uk/showcase/). All the participants gave their consent.
Details of the Olink proteomics assay, data processing and quality control are provided in the Supplementary Information. One protein (GLIPR1) had >80% of data failing quality control (99.4% failing quality control; Supplementary Table 3) and was excluded from analyses. We didn’t perform any further NPX processing after learning of the quality-control procedures. Each protein level was inverse-rank normalized, including NPX data below the LOD, before analyses and association testing.
We estimated the association of proteins levels with quantitative traits using linear regression. Logistic regression was used to estimate the association ofprotein levels with prior or past disease. All analyses were adjusted for the sex and age of the person at the time of collection.
The UKB-PPP data was randomly subsetted into 80% of the models for training. The Abo blood group and FUT2 secretor status analysis is described in the section, along with age, sex, BMI,AST and eGFR, as well as genetic ascertainment of blood groups. The same genes are measured (AST) or used in the production of eGFR (cystatin C) so we excluded them. Performance was evaluated in the held out 20% test data. The remaining of missing measurement were subtracted from the total in the predictor models.
We found all the variants in NHGRI-EBI’s catalog of human Gwas74 that were reported in highLD with pQTLs from Olink-UKB-BI data. For each sentinel pQTL association, we also identified a 95% credible set of variants (variants that most parsimoniously explain regional association75) likely to include the causal variant76. We then checked whether GWAS catalogue variants in high LD with the pQTL variant (with r2 > 0.8 with the pQTL) were included in the credible set. The highlighted examples included high LD between disease-associated and both pqTL and variant in the credible set, and we estimated the odds of colocalization when they were not identical.
Individual protein levels (NPX) were inverse-rank normalized before analysis including NPX data below the LOD. The discovery cohort association models included age, age2, sex, age sex, age2 sex and the first 20 genetic principal components. The covariates in the replication and full cohort along with genetic ancestry-specific analyses also included if the person was preselected for the COVID-19 study.
We used a conservative multiple-comparison-corrected threshold of P < 1.7 × 10−11 (5 × 10−8 adjusted for 2,923 unique proteins) to define significance. We defined primary associations through clumping ±1 Mb around the significant variants using PLINK60, excluding the HLA region (chromosome 6: 25.5–34.0 Mb), which is treated as one locus owing to complex and extensive LD patterns. The lowest P value variant was deemed the primary associated variant after the merging of overlapping regions. We use the most significant association and the primary associations that overlap with the major marginal associations to make a list of the regions associated with multiple proteins. In the cases in which the primary associations were marginal, we grouped them together iteratively until convergence.
Annotation was performed using Ensembl Variant Effect Predictor (VEP), WGS Annotator (WGSA) and UCSC Genome Browser’s variant annotation integrator (http://genome.ucsc.edu/cgi-bin/hgVai). The gene/protein consequence was based on RefSeq and Ensembl. We reported exon and intron numbers that a variant falls in as in the canonical transcripts. For synonymous mutations, we estimated the rank of genic intolerance and consequent susceptibility to disease based on the ratio of loss of function. For coding variants, SIFT and PolyPhen scores for changes to protein sequence were estimated. The Encyclopedia of DNA Elements Project mapped histone marks and open chromatin areas for non-coding variants. For intergenic variants, we mapped the 5′ and 3′ nearby protein-coding genes and provided distance (from the 5′ transcription start site of a protein-coding gene) to the variant. The score for non-coding versions was estimated. An enrichment analysis hypergeometric test was performed to estimate enrichment of the associated pQTL variants in specific consequence or regulatory genomic regions.
We used the sum of single-effects regression to find and fine-map independent signals using individual-level data. Our inputs for SuSiE were mean-centred and unit variance genotype and phenotype residuals accounting for the same covariates as for the marginal association analysis. We took the residuals from the phenotype for polygenic effects and sample relatedness.
We performed two sample randomizations to estimate the effect of PCSK9 abundance on genetic liability. We estimated the effects for each individual variant using the two-term Taylor series expansion of the Wald ratio and the weighted delta inverse-variance weighted method to meta-analyse the individual SNP effects to estimate the combined effect of the Wald ratios. Standard sensitivity analyses were used to analyse the results of the randomization analyses. Evidence of how the estimated effect was oriented from PCSK9 abundance to the outcome and not due to reverse causality was provided using Steiger filtering.
The tissues were enriched with the genes from the Olink panel using the TissueEnrich R package. For enrichment of human genes, we used the data from the HumanProtein Atlas 70 with all genes that were found to be expressed within each tissue and for orthologous mouse genes, we used data from a previous study. For multiple comparisons, the enrichment P-value thresholds were adjusted based on the number of tissues tested where applicable.
The blood group is imputed by using the blood type imputation method in the UKB. FUT2 secretor status was determined by the inactivating mutation (rs601338), with genotypes GG or GA as secretors and AA as non-secretors. The relationship between blood group and secretor status was tested for the same covariates as in the major pQTL analyses. A multiple-testing threshold of P < 1.7 × 10−5 (0.05/2,923 proteins) for the interaction terms was used to define statistically significant interaction effects.
Multi-trait colocalization in the COVID-19 Host Genetics Consortium: Effect of season and fasting time on variant association with amylase levels
The COVID-19 Host Genetics Consortium updated the top loci for colocalization with their estimates from the R7 summary results. A region association threshold of 0.8 is the minimum threshold we used to perform multi-trait colocalization.
The effect of the season and the amount of time fasted at blood collection on variant associations withamylase levels were reanalyzed. Blood collection season (summer/autumn (June to November) versus winter/spring (December to May)) was defined on the basis of the blood collection date and time (field: 3166). Participant-reported fasting time was derived from field 74 and was standardized (Z-score transformation) before analysis.
Blood cell composition was further tested for association of genes with blood cell measure and not in the sensitivity analyses. The first thing we did was identify the blood cell phenotypes that were linked to P 1.7 1011 within a multivariable linear regression model and adjusted for other variables. Before testing for mediation, we were able to confirm that there was an association between the genetic variant and the blood cell phenotypes. In the final test, we compared the strength of associations, genotype → protein, to that of the genotype → protein in a multivariable model (protein ~ dosage + blood cell phenotype + discovery covariates) to establish whether the variant–protein association is either fully (P > 0.01) or partially (P < 1.7 × 10−11) mediated by the blood cell phenotype.
We clumped the parameters of PLINK with the first step of the experiment to create test regions that accounted for potential long-range LD. For each clump, we extended the coordinates of the left- and right-most variants to a minimum size of 1 Mb, merged overlapping clumps and defined these as the test regions.
Each test region was tested using the parameters min_abs_corr and L_ max_iter. For test regions in which SuSiE found the maximum number of independent credible sets, which was initially set at L = 10, we incremented L by 1 until no additional credible sets were detected. A filter was applied to remove credible sets from high LD and another in the same region (lead variant r2 > 0.8). For regions with more than one credible set, we assessed statistical independence by performing multiple linear regression with most probable variations for each set and the same phenotype and genotype residuals.
If the pQTL is located within 1 billion of the start site for the gene, then its association will be cis.
It was possible to identify samples likely to be labeled incorrect by using individual predictions of sex, and of the levels of proteins in the sample. Whole plates or individual rows or columns of samples, identified as being majority likely incorrectly labelled, were excluded from the UKB-PPP data. In a total of 1,179 samples, 13 whole plates, five rows or columns of samples were excluded from the Expansion set of assays. There were four whole plates and seven row or column of samples that were left out of the 1536 set of assays. In the 1536 set of assays, one panel was excluded for two plates and affected 174 samples.
We used health care records to make lists of disease diagnoses. This resulted in 275 case–control phenotypes. We had scores from various sources but not at the same time as the sample was gathered.
Probing the ratio of observed to expected CV of repeated measurements in GWAS, GTEx, and Human Protein Atlas using real-time genomic tools
We suggest you consider the CV of repeated measurements relative to the expected CV if the samples are not of the same sample, but of samples selected from the population. The ratio of s.d. to this metric is the same as the ratio of all the measurements. A CV ratio of 0 requires the s.d. of repeated measurements to be 0, indicating that they are fully correlated and therefore that the precision is 100%. The CV ratio requires the s.d. of measurement repeat to be the same as the random measurement, which is not correlated and shows the precision is zero. We evaluated the platforms based on the ratio of observed CV to expected CV, if the measurements were independent.
The GWAS catalog, the GTEx project and the Human Protein Atlas are external data that can be used.
The software we used is publicly available. BamQC (v1.0.0, https://github.com/DecodeGenetics/BamQC), GraphTyper (v2.7.1, v1.4, v2.7.2, https://github.com/DecodeGenetics/graphtyper), GATK resource bundle (v4.0.12, gs://genomics-public-data/resources/broad/hg38/v0), Svimmer (v0.1, https://github.com/DecodeGenetics/svimmer), popSTR (v2.0, https://github.com/DecodeGenetics/popSTR), Admixture (v1.3.0, https://dalexander.github.io/admixture), Dipcall (v0.1, https://github.com/lh3/dipcall), RTG Tools (v3.8.4, https://github.com/RealTimeGenomics/rtg-tools), bcl2fastq (v2.20.0.422, https://support.illumina.com/sequencing/sequencing_software/bcl2fastq-conversion-software.html), Samtools (v1.9, v1.3, https://github.com/samtools/samtools), samblaster (v0.1.24, https://github.com/GregoryFaust/samblaster), BWA (v0.7.10 mem, https://github.com/lh3/bwa), GenomeAnalysisTKLite (v2.3.9, https://github.com/broadgsa/gatk), Picard tools (v1.117, https://broadinstitute.github.io/picard), Bedtools (v2.25.0-76-g5e7c696z, https://github.com/arq5x/bedtools2), Variant Effect Predictor (release 100, https://github.com/Ensembl/ensembl-vep), BOLT-LMM (v2.1, https://data.broadinstitute.org/alkesgroup/BOLT-LMM/downloads), IMPUTE2 (v2.3.1, https://mathgen.stats.ox.ac.uk/impute/impute_v2.html), dbSNP (v140, https://www.ncbi.nlm.nih.gov/SNP), BiNGO (v3.0.3, https://www.psb.ugent.be/cbd/papers/BiNGO/Download.html), Cytoscape (v3.7.1, https://cytoscape.org/download.html), COLOC (v5.1.0.1, https://github.com/chr1swallace/coloc). The genomics and pQTL processing pipelines have been extensively described previously2,12. Olink Explore is a program we used to process data generated on the Olink platform. Data was analysed and figures were generated using a number of packages.
It is possible to create a barcode with the Olink Explore 3072 proximity extension kit by binding two polyclonar antibody pools to a target and then having two single-stranded DNA probes combine with each other. The platform consists of 2,941 immunoassays targeting 2,925 proteins. Each assay is based on a pair of polyclonal antibodies. The Antibody are labeled with a single strand of the oligonucleotide that they bind to. If matching pairs of antibodies bind to the protein, the attached oligonucleotides hybridize, and are then measured using next-generation sequencing59,60. Olink Explore 3072 has 8 panels of assays analysed. Olink Explore 1536 is a subset of the Olink Explore 3072 and includes the Expansion set. The Olink values were based on the NPX values recommended by the manufacturer.
In UKB, we used health care records to identify a disease category by the first three letters of the corresponding ICD10 code. When the number of people diagnosed exceeded 50, we were able to figure out the correlation between their genetics and their disease diagnosis. This resulted in 324, 29 and 20 case–control phenotypes for UKB-BI, UKB-AF and UKB-SA, respectively. In addition, we had measurements of 208, 56, and 60 quantitative traits in UKB-BI, UKB-AF and UKB-SA respectively with at least 50 individuals measured for each trait. The quantitative traits were measured at the same time as the plasma was collected, when available.
Both Olink and SomaScan use dilutions of plasma samples to compensate for different concentrations of proteins in plasma13,59,62. The two platforms agree on the placement of the set of protein into low, intermediate and high groups.
Source: Large-scale plasma proteomics comparisons through genetics and disease associations
A comparison of Olink and SomaScan with different platforms for quality control of the synthesis of protein levels and comparing accuracy of biomarkers
Accuracy refers to how similar repeated measurements will be while CV is the s.d. of measurements divided by their mean. CV is not always a good measure of accuracy. A platform with a low CV, but no accuracy, might be able to produce random values in a tight range.
To compare the accuracy of the assays, we assumed the two duplicates were not independent of each other, and adjusted the CV to account for the available duplicate measurements. The estimate of the sd. of the repeated measures was inserted into the formula for the CV above, obtaining CVrep. Note that CVrand is the same as the CV of all protein levels and that the ratio CVrep/CVrand corresponds to the ratio of the s.d. of the repeated measurements to the s.d. of all protein levels, and will be expected to be 0 if the repeats are always the same and 1 if they are independent of each other (Fig. 1, Supplementary Fig. 1 and Supplementary Tables 1 and 2).
Both Olink and SomaScan use control samples specific to the platform for quality control. The evaluation does not include the inter-plate variation if only two measurements of the same control sample are used to evaluate the CV. Comparing the CV ratio computed from the repeated control samples between the two platforms can therefore help comparison in terms of batch effects, with values closer to one suggesting that the platform is less susceptible to batch effects and closer to zero that the platform is more so (Supplementary Note 2, Supplementary Fig. 9).
We used a likelihood ratio test to calculate P values and then adjusted them for multiple testing using the same significance threshold as we used on the previous study.
Over half of the variants with cis pQTL and almost all of the variants with trans pQTL are in high LD with a PAV over 0.80.
The 0.05 P value threshold is used for replication between platforms, it requires that initial and replicated associations are in the same direction.
Source: Large-scale plasma proteomics comparisons through genetics and disease associations
Detecting pQTLs in a large-scale plasma proteomics comparison between transgeneous and major histocompatibility regions
We considered sequence variants from the conditional analysis to belong to the same region if they were within 2 Mb of each other. Furthermore, we considered the major histocompatibility complex (MHC) region (build 38 chr. 6:25.5-34.0MB) as a single region. We refer to the most significant variant in each region as the sentinel variant for the assay in the region, and other variants as secondary variants.
We used theLD based clumping approach to identify pQTLs associated with multiple assays and if they are in high LD, to belong to the same pQTL.
There is a high chance of rejecting the null hypothesis of no association if the P value threshold is P, sample size N, effect size, and MAF f.
We tested to see if the variant itself and the variant in high LD could affect the coding sequence of genes.
When considering the neighboring genes within 1 MB we discover that cis pQTLs are more likely than trans to have a PAV on both platforms. The results were the same on both platforms.
The same approach that Sun et al.6 used to determine the location of the cytoplasm was used to determine the location of the subcellular structures.
Source: Large-scale plasma proteomics comparisons through genetics and disease associations
Analysis of Anti-NF-Light Immunocapture Complexes of Plasma Samples on a Quantitative SR-X Instrument
Blood was collected in EDTA tubes that were inverted 4–5 times and then centrifuged for 10 min at 3,000g at 4 °C. Plasma samples were frozen in aliquots at −80 °C. Plasma aliquots were allowed to thaw on ice and kept away from light during defrosting. Before measuring, the aliquots were mixed with the tubes by inverting them a few times and then placed in a tank for 10 minutes at 4 C.
Plasma samples were measured in duplicates with commercially available Simoa NF-light Advantage (SR-X) kit (Quanterix, cat. 103400). Samples were diluted 4:1 and incubated with 25 µl anti-NF-light immunocapture beads and 20 µl biotinylated detector antibody at 30 °C and 800 rpm for 30 min. The bead-immuno complexes were washed and resuspended and then put into a culture dish of 100 l and 800rpm for 10 minutes. After a second washing step, the bead-immunocomplexes and resorufin β-d-galactopyranoside were loaded onto an SR-X instrument (Quanterix) for processing and analysis.
To determine the colocalization of coronavirus variants, we identified a 95% credible set of variants likely to include the causal variant76. We identified primary associations through clumping 1 Mb around the significant variants using PLINK60. An enrichment analysis hypergeometric test was performed to estimate enrichment of the associated pQTL variants in specific consequence or regulatory genomic regions.